By Leah Mann
THE IDEA
A team led by Vanderbilt researchers have developed an active machine learning approach to predict the effects of tumor variants of unknown significance, or VUS, on sensitivity to chemotherapy. VUS, mutated bits of DNA with unknown impacts on cancer risk, are constantly being identified. The growing number of rare VUS makes it imperative for scientists to analyze them and determine the kind of cancer risk they impart.
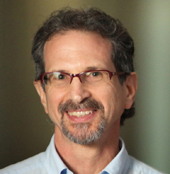
Traditional prediction methods display limited power and accuracy for rare VUS. Even machine learning, an artificial intelligence tool that leverages data to “learn” and boost performance, falls short when classifying some VUS. Recent work by the labs of Walter Chazin, Chancellor’s Chair in Medicine and professor of biochemistry and chemistry, and former Vanderbilt professor Tony Capra, now at the University of California, San Francisco, led by co-first authors and postdoctoral fellows Alexandra Blee and Bian Li, developed an active machine learning technique to address the problem.
Active machine learning relies on training an algorithm with existing data, as with machine learning, and feeding it new information between rounds of training. Chazin and his lab identified VUS for which predictions were least certain, performed biochemical experiments on those VUS and incorporated the resulting data into subsequent rounds of algorithm training. This allowed the model to continuously improve its VUS classification.
The researchers validated their approach on four proteins known to be implicated in cancer. With a validated algorithm in hand, they applied it to uncharacterized VUS involved in a DNA repair pathway called NER—mutations in DNA repair pathways are frequently associated with cancers—and demonstrated that active machine learning could better predict the variants’ effects on protein function and chemotherapy sensitivity compared with traditional machine learning.
WHY IT MATTERS
Although rare VUS identified in tumor genomes are unlikely to be primarily responsible for the initial development of those tumors, they may nevertheless impact tumor growth and response to therapy. Characterizing VUS can optimize clinical care, and adding active learning frameworks to the VUS-interpretation toolkit can improve clinicians’ ability to employ precision medicine for each patient.
WHAT’S NEXT
This work lays the foundation for studies on the mechanisms underlying the dysfunction and chemotherapeutic response of cells expressing certain VUS involved in NER.
The Chazin lab and their collaborators Capra and Zachary Nagel at Harvard University—will focus on updating the algorithmic framework and adding to data to improve its predictive power.
FUNDING
This work was funded by the National Institutes of Health, the American Heart Association and the Humboldt Professorship of the Alexander von Humboldt Foundation.
GO DEEPER
The article “An Active Learning Framework Improves Tumor Variant Interpretation” was published in Cancer Research on Aug. 1.