By Jenna Somers
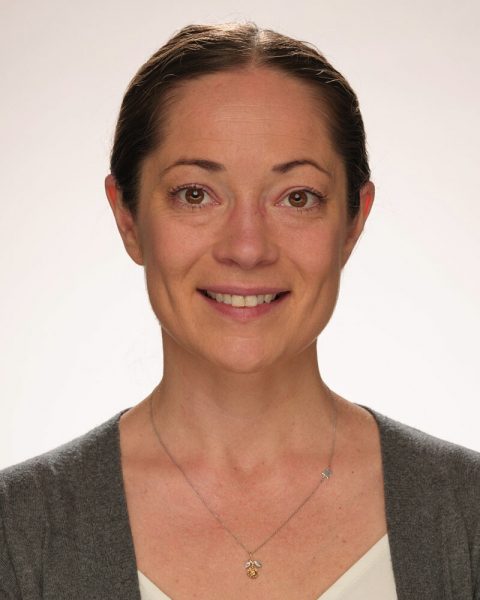
A three-year, $1.6 million grant from the National Institute of Child Health and Human Development will support Sophia Vinci-Booher, assistant professor of psychology and human development at Vanderbilt Peabody College of education and human development, and her team in evaluating brain development for letter and number learning in early childhood, specifically how brain responses for letters and numbers emerge and develop throughout the first grade. Vinci-Booher’s novel approach to measuring brain activity could improve learning interventions and advance the development of artificial intelligence to capture human learning in early childhood.
The first grade is a year of foundational learning that sets the stage for reading and math outcomes in the later elementary school years when falling behind leaves children more likely to experience economic and medical instability as adults. Despite these potential negative public health and social outcomes, young children are under sampled in neuroimaging research. As a result, developmental scientists do not know the brain changes that unfold during this foundational year of learning; yet this knowledge could lend valuable insights related to learning intervention.
That’s according to Vinci-Booher, the principal investigator on a multi-institutional study examining brain function for child learning via a novel approach of multiple MRI measurements of brain activity during a relatively short window of learning. Vinci-Booher refers to this approach as dense longitudinal neuroimaging and says it has never been done before. Importantly, her pathbreaking research has the potential to transform understanding of human learning by capturing how the brain changes during foundational learning.
“Developmental scientists often focus on process. We want to capture the ups and downs – the waxing and waning – of how a behavior progresses over time. This will be the first neuroimaging study designed to capture the process of learning in the brain. Understanding process in the brain leads to effective interventions, not only because we know more about how the brain should change over time, but also because we can identify timepoints at which the brain is likely to be most sensitive to intervention,” Vinci-Booher said.
The project brings together a highly collaborative team with expertise that spans multiple scientific domains, including not only development and neuroimaging but also vision sciences, computational modeling, and informatics. In addition to PI Vinci-Booher, the team includes James Booth, Patricia and Rodes Hart Professor of Educational Neuroscience at Peabody College, Kendrick Kay, assistant professor of radiology at the University of Minnesota; Franco Pestilli, associate professor of psychology, and Chen Yu, Charles and Sarah Seay Regents Professor of Psychology, both at the University of Texas at Austin.
Vinci-Booher and her team will derive brain responses associated with letters and numbers from first graders who will, monthly, view Sesame Street Images while MRI measures their brain activity. The Sesame Street Images will be taken from a corpus of 35,000 Sesame Street Images that the research team will create and distribute to the scientific community for reuse. Each Sesame Street image will include annotations for educational content, including letters, numbers, and other objects that will allow the research team to derive brain responses associated with letter and number processing. The frequency of the scans will create a dense longitudinal MRI dataset that the research team will use to evaluate brain changes throughout the first year of schooling.
Designing AI to replicate human learning
This study will advance computational modeling of human learning, which could lead to new knowledge on how, why, and when humans learn. The team will share the Sesame Street Images and the Sesame Street MRI dataset with the scientific community for reuse – two resources that will be of great value for the design of AI to capture human learning in early childhood.
The Sesame Street Image Corpus will be the first large-scale image dataset that will allow AI to train on visual scenes of typical child educational materials. AI that trains first on child data, to learn as children learn, is likely to replicate human learning more authentically than current training procedures that use only adult data. Furthermore, AI training can use the Sesame Street MRI Dataset to incorporate experimentally measured brain responses to further enhance understanding of human learning.
Ultimately, this research could lead to pivotal advancements in human and artificial learning that spans several fields, including cognitive, developmental, and educational neuroscience as well as neuroimaging methods, computer vision, and learning sciences.