Vanderbilt University School of Nursing Assistant Professor Alvin Jeffery is a biomedical informatics expert, lending his expertise in developing back-end computational work to problems across health care. Here, Jeffery details studies funded by two recently awarded grants, including a grant of more than $2.3 million over five years from the National Institute on Drug Abuse.
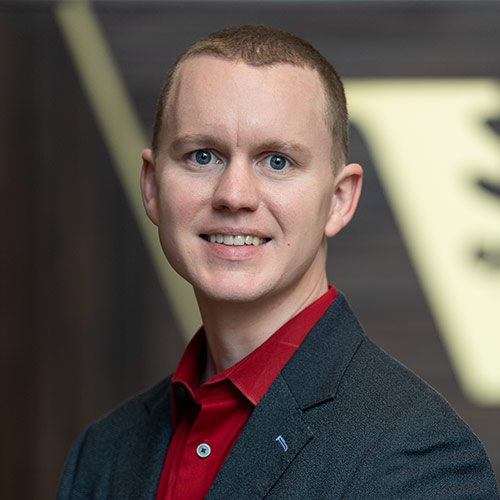
How are your current projects helping you grow as a researcher?
The first is funded by a Betty Irene Moore Fellowship for Nurse Leaders and Innovators, to evaluate the technology nurses and other clinicians use to deliver better care. There’s a great deal of complex quantitative information bombarding clinicians these days, and I think a big reason why some advanced tools haven’t helped patients is because they’re not delivered in ways that are responsive to how health care workflows function. With the grant, we’re developing a method that allows us to test different ways of displaying information and hopefully converge on solutions that are more meaningful. The grant also has a big focus on my leadership skills. This is an excellent opportunity to merge my research and leadership skills because I know that without good communication, leadership, team management and professional networking, even the best ideas may not be translated to get to the people that need them.
The second is a $2.3 million, five-year grant from the National Institute on Drug Abuse. Our work will focus on developing technology that can identify through the electronic health record people who are likely to have substance use disorder, with the specific application of supporting genetic research across multiple organizations. Opioid and substance use disorders are not well documented, which gives geneticists a lot of trouble conducting research at the scale that they want to. I’m new to studying substance use disorders and genetics, but I’m excited to apply my informatics and clinical backgrounds to the work of creating a framework that allows health care providers in any organization to develop their own definition of substance use disorder—and then leverage data collected by multiple organizations to compare them equally across the different organizations.
A lot of my work has been centered around finding nursing-sensitive outcomes, which are not always well-described in the structured data of the electronic health record. Over time I’ve developed data science methods and informatics approaches that allow me to play with data in unique ways. To conduct our work, my team works with Vanderbilt’s mighty Advanced Computing Center for Research and Education. As a result of the NIDA grant, I’m able to add machine learning scientists, computational linguists, statisticians, data scientists, software developers and geneticists to my team.
What is the role of geneticists and genetics in substance use disorder treatment?
There are two ways that we think genetics might play a role in helping people: identifying those at risk and personalizing management for people with substance use disorders. We are approaching this by looking at several potential genetic profiles. Let’s say somebody has surgery, and they get prescribed pain medication such as narcotics or opioids. What if they have a genetic risk or a genetic makeup that makes them more susceptible to developing an opioid or substance use disorder? We might manage their post-surgical care differently if we know ahead of time that they’re at risk for developing a problem. On the other end of the spectrum, if we are working with those who do have a substance use disorder, they might benefit from different medications or different pain management approaches based on their genetic makeup.
The way that we’re thinking about our data and the outcome of our work is not using data to definitively conclude that a patient has or does not have substance use disorder, but rather falls along a continuum. People may move from a little bit of dependence to a worrisome dependence on a substance without it interfering with daily life, but where could a person fall? Perhaps we need different treatments at different moments along the way. Recognizing that substance and opioid use disorder is a continuum hasn’t really been done.
Why did you focus your informatics work in this area?
Theologian and author Frederick Buechner said our calling is where our great desires and strengths meet a deep need in the world. My start in research was predicting which hospital patients were going to deteriorate and getting that information to clinicians in ways that make sense to them. Along the way, I developed these data science skills to find these unknowable outcomes, and I happened to do that with a specific use case of opioid induced respiratory depression—a biological or genetic condition where opioids desensitize the brain stem to increases in carbon dioxide, which can rapidly lead a patient to stop breathing after surgery. Geneticists in the substance use disorder space looked at the work and said, “Wait, you have a skill that we’ve been missing in our field.” As soon as we partnered, we found a wonderful synergy.
And there is definitely a deep need in the world, in particular the United States, to address substance use disorder. One in 12 adults in the U.S. have a substance use disorder problem of some sort. Responding to this crisis costs over $600 billion annually. It’s one of many big problems, and it’s where my strengths line up well. I love that this project is one small piece of the larger legacy that I’m developing over my career, which is making the right information available at the right time to help clinicians make the best decisions for and with patients and their families.
How are you planning to pull together the sheer amount of data you’re working with and have it make sense to more users?
With my Vanderbilt, Vanderbilt University Medical Center and VA appointments, I have access to lots of data that is helping my team build this framework. At the end of the day, we think we will pull together two or three million people to do these genetic studies, which is where we really want to be in terms of confidence in genetic association studies.
As far as harmonizing, let’s say we’re trying to share an algorithm to find opioid use disorder between the VA and Vanderbilt. One thing about the VA is that most of their patients get their prescriptions filled in their pharmacy. So, we know not only that a patient got a prescription for something, but they also filled it and picked that medication up. If someone has a prescription for Suboxone, which is used to treat opioid use disorder, you can pretty much guarantee that they have an opioid use disorder, even if they don’t have a diagnosis for it. If the VA builds their algorithm to say if a patient got a Suboxone prescription filled, then they have this disorder. We can’t transfer that to Vanderbilt because not all of Vanderbilt’s patients use Vanderbilt’s pharmacy. The method that we’re proposing is instead of saying definitively, “yes, they have it” or “no, they don’t” is to offer the probability that that patient has that condition of interest.
This probability can be constructed based on data like whether or not the patient got Suboxone as well as other things. If they got a diagnosis or if they have a drug screen that says they tested positive for opioids, that increases the probability. Some of those rules could be part of an algorithm that Vanderbilt adopts, and the probabilities may be less certain, but it could be a probability nonetheless. And so even though one of the variables is missing, like the pharmacy data, we could still get a probability. This also has the benefit of letting the data scientists nerd out a little bit in terms of genetic sensitivity by training the models to find a signal within all that noise.
What happens after a patient’s probability for opioid or substance use disorder is determined?
Say the patient has a 78.3 percent chance of having a substance use disorder. We feed that into our genetic analysis software. Based on what we see in the data, we determine the modifications, abnormalities or differences in the patient’s genetic structure making them more at risk for a substance use disorder. If we get into treatment, we may put a different outcome variable to say that this genetic composition is more likely to respond to treatment A compared with treatment B. The big difference is that how we define substance use disorder and how we represent those people in the data that the computer analyzes is going to be a lot different.
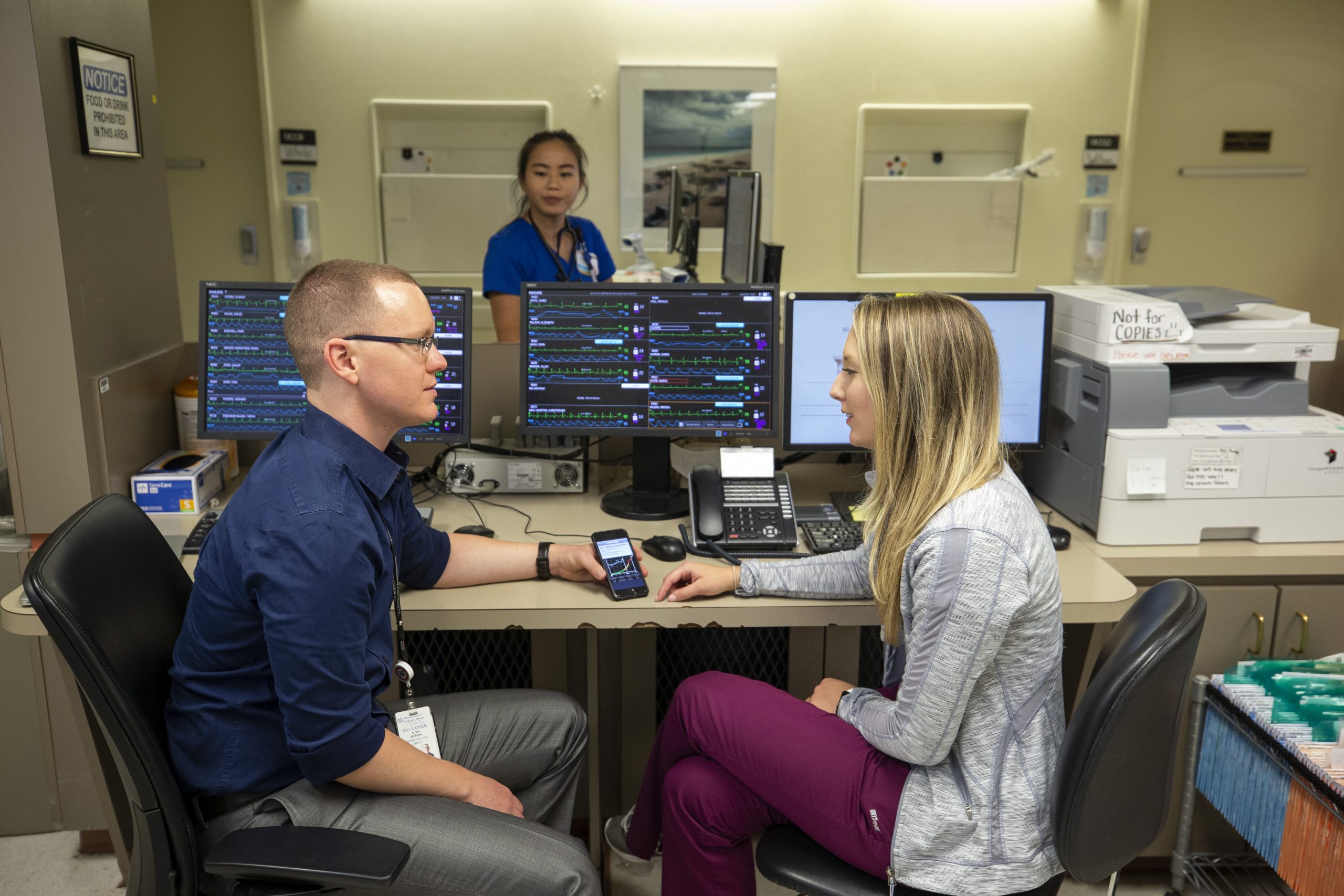
What’s unique about Vanderbilt that makes this work possible?
It’s so helpful that I trained at Vanderbilt, VUMC and the VA and that the School of Nursing allowed me to retain my VA appointment. Not every VA and academic partner has this amount of crossover and collaboration—sometimes to where you don’t even know where someone’s funding comes from. Having access to multiple organizations allows my team to test nearly any hypothesis and to conduct research that we couldn’t with access to only one partner. That Vanderbilt was willing to take a chance on this approach is a major element that allowed me to receive this NIDA grant.
What are the long-term implications for this work?
The software we’re developing will lend itself to use surrounding other psychiatric and mental health disorders, many of them manageable if not curable, but at least manageable if we can destigmatize them.
I can imagine a time when we are able to expand this approach beyond substance use disorders to depression, schizophrenia, anxiety, bipolar disorder. That may help people and communities as we put more quantifiable, empirical, biological, mechanistic approaches behind treatment options and ultimately begin to destigmatize mental health disorders.