Vanderbilt and VUMC researchers have created a technique that corrects distortions in MRI images, which helps researchers and radiologists to better interpret brain scans.
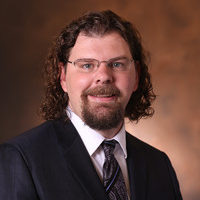
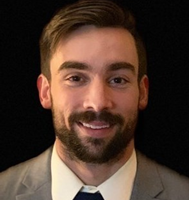
The work by Bennett Landman, professor of electrical engineering and computer science and radiology and radiological sciences, and Kurt Schilling, research assistant professor of radiology and radiological sciences was published online in the article, “Distortion correction of diffusion weighted MRI without reverse phase-encoding scans or field-maps” in the journal PLoS ONE on July 31.
“To quantify anything in the brain is highly important,” said Landman, the project’s lead researcher. “Incorrect images can distort the image’s intensity, understanding of brain size volume or interpretation of connections of brain pathways. If we don’t have a true image, we cannot accurately observe or describe brain connections, which will negatively affect neurological research.”
Distorted images are common—an image of a three-dimensional object will get squashed or pulled in ways that don’t reflect what the object truly looks like—but this is especially important to fix when the image is of a brain and its purpose is to understand disease or disorder. The new algorithm, Synb0-DisCo, developed by the core faculty at the Vanderbilt Institute for Surgery and Engineering and the Vanderbilt University Institute of Imaging Science, synthesizes what the MRI image should look like from anatomically correct images and uses that data to correct the MRI scan that was acquired.
The analyzed datasets—thousands of images to date—include legacy images from the Human Connectome Project, a large-scale National Institutes of Health-funded project that constructed a complete map of the structural and functional neural connections of the brain, the Autism Brain Imaging Data Exchange and the Baltimore Longitudinal Study of Aging, the largest effort to understand how the brain develops in aging. Images from projects like these are used by tens of thousands of researchers around the world studying the human brain.
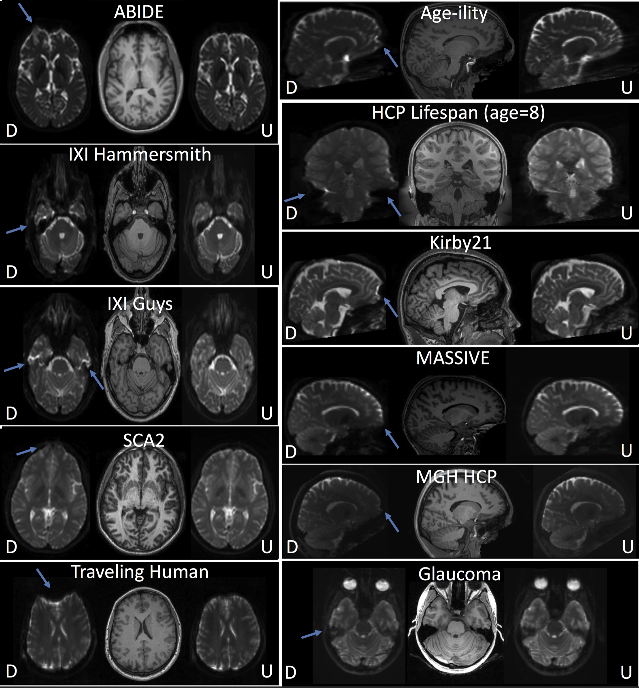
“We’ve been able to use deep learning to synthesize contrasts,” said Schilling, the paper’s lead author. “The idea of using information from huge datasets and applying it to a single dataset was a black box before we developed this technique. We’ve been able to learn things about the brain that we never would have been able to, working with only our datasets. That has been a remarkable outcome of this work.”
In clinical settings, diffusion MRI scans are used preoperatively and during procedures for the surgical removal of tumors. In both settings, it is crucial to ensure that images are geometrically sound. Distorted images most significantly affect stroke patients. Synb0-DisCo has been very positively received by the research community, with one researcher indicating that since finding the algorithm they have been able to pick up projects that otherwise would have been abandoned and another recognizing the algorithm’s potential and impressiveness.
This work was funded by the National Institutes of Health grants R01EB017230 and T32EB001628, by the National Center for Research Resources grant UL1 RR024975-01 and Department of Defense award W81XWH-17-2-055.