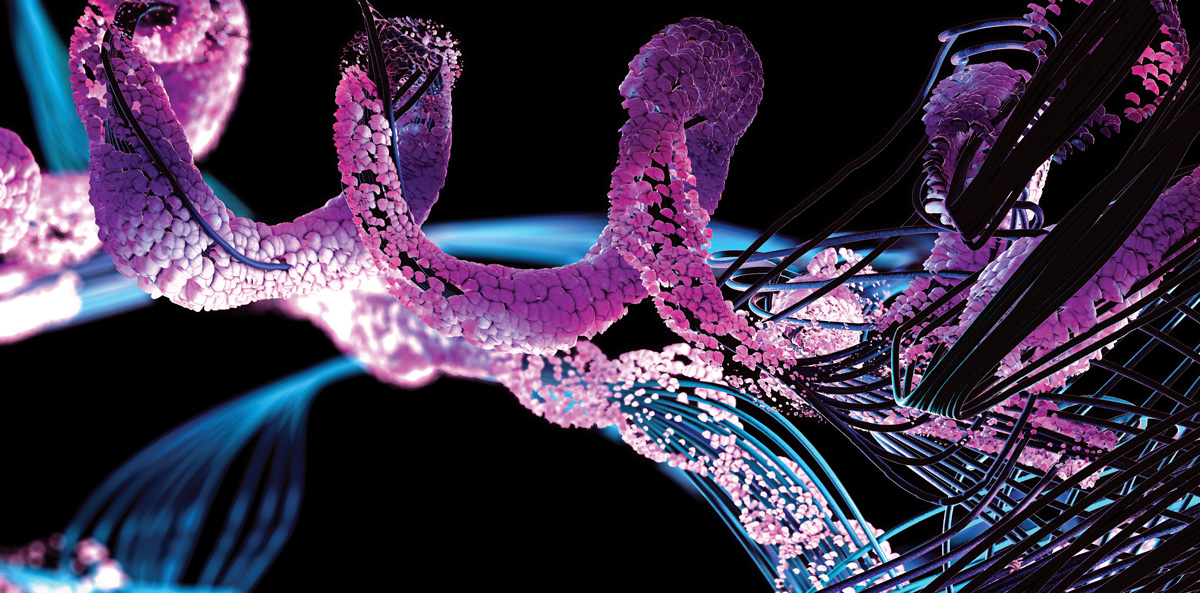
It’s one thing to know what a protein looks like on paper—it’s another to know how it looks in real life.
The “protein folding problem” has been one of the most vexing in biology. Even if a scientist knows the DNA sequence for a protein, it’s virtually impossible to predict how the long chains of amino acids will interact and fold in upon themselves to create a three-dimensional model.
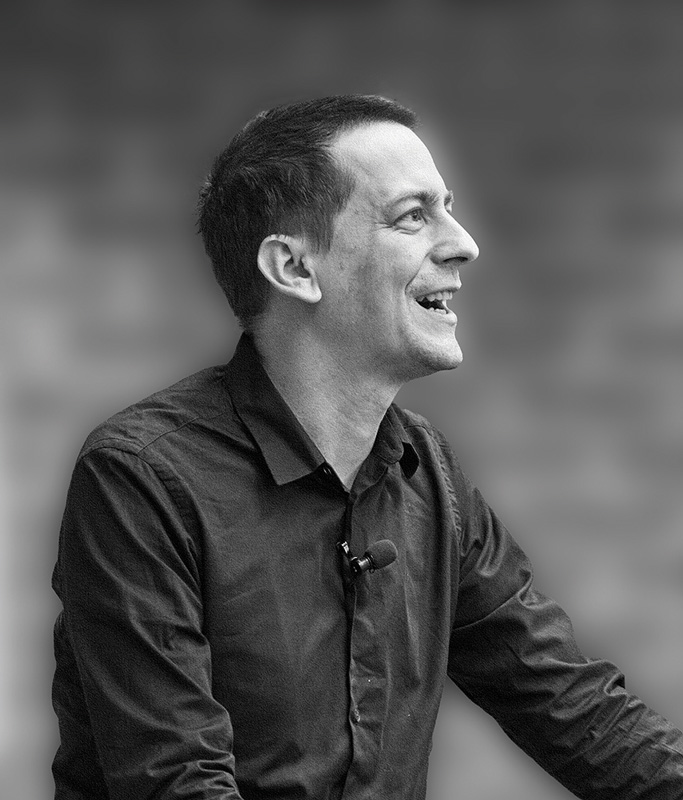
“The genome gives us the wrong view of proteins—it gives us a linear view,” John Jumper, BS’07, said at the Apex Lecture, a series sponsored by the Vanderbilt School of Medicine Basic Sciences this past August. You might see several mutations associated with cancer spread out along the chain “and then you look at the structure, and they are all right next to each other,” he said. Such particularities are essential in understanding how a protein functions and how it might be targeted. “It gives us a great tool for making hypotheses about how the cell works that we can ultimately test.”
Jumper is a senior staff research scientist for DeepMind, a London-based company that made a huge leap forward in solving the protein folding problem using artificial intelligence. It first released its prediction software, AlphaFold, in 2018, following it up with the even more accurate AlphaFold 2 in late 2020. The method has produced extremely accurate 3D models of all 200 million or so proteins known to science—all freely available on the cloud. In a competition, it outperformed all other methods of protein structure prediction, including those that are much more labor intensive and time-consuming. The work is so significant that Jumper was awarded the 2023 Albert Lasker Award for Basic Medical Research, which is often considered a precursor prize to the Nobel.
The implications of AlphaFold’s work are vast, allowing for quick, cost-effective predictions that let scientists custom-design drugs to target certain proteins involved in disease, design synthetic enzymes for chemical reactions, speed production of new vaccines and even personalize medical treatments. “AlphaFold represents a revolutionary advance in structural biology, one that has brought the holy grail of predictive protein folding into the toolkit of biochemistry and molecular biology,” said John Kuriyan, dean of the School of Medicine Basic Sciences, in announcing Jumper’s selection for the Apex Lecture, which focuses on breakthroughs in biomedical science. “The impact of these advances on drug discovery and, ultimately, on human health will be enormous.”
At the lecture, Jumper took a deep dive into how he and his fellow scientists trained a neural network on the Protein Database (PDB) to create the model that became AlphaFold. There was no magic bullet, he said. Rather, researchers painstakingly trained the model on pairs and sequences of amino acids using various techniques, knocked out particular genes to observe effects, and even asked the model to critique itself on the road to ever more accurate predictions. “We had many small effects that we were able to put together and many, many ways in which we got a little bit better,” Jumper said. “We found that everything mattered a bit, and nothing mattered a lot.”
— MICHAEL BLANDING